Identification of the Optimal Location of Co-Working Spaces using Clustering Methods
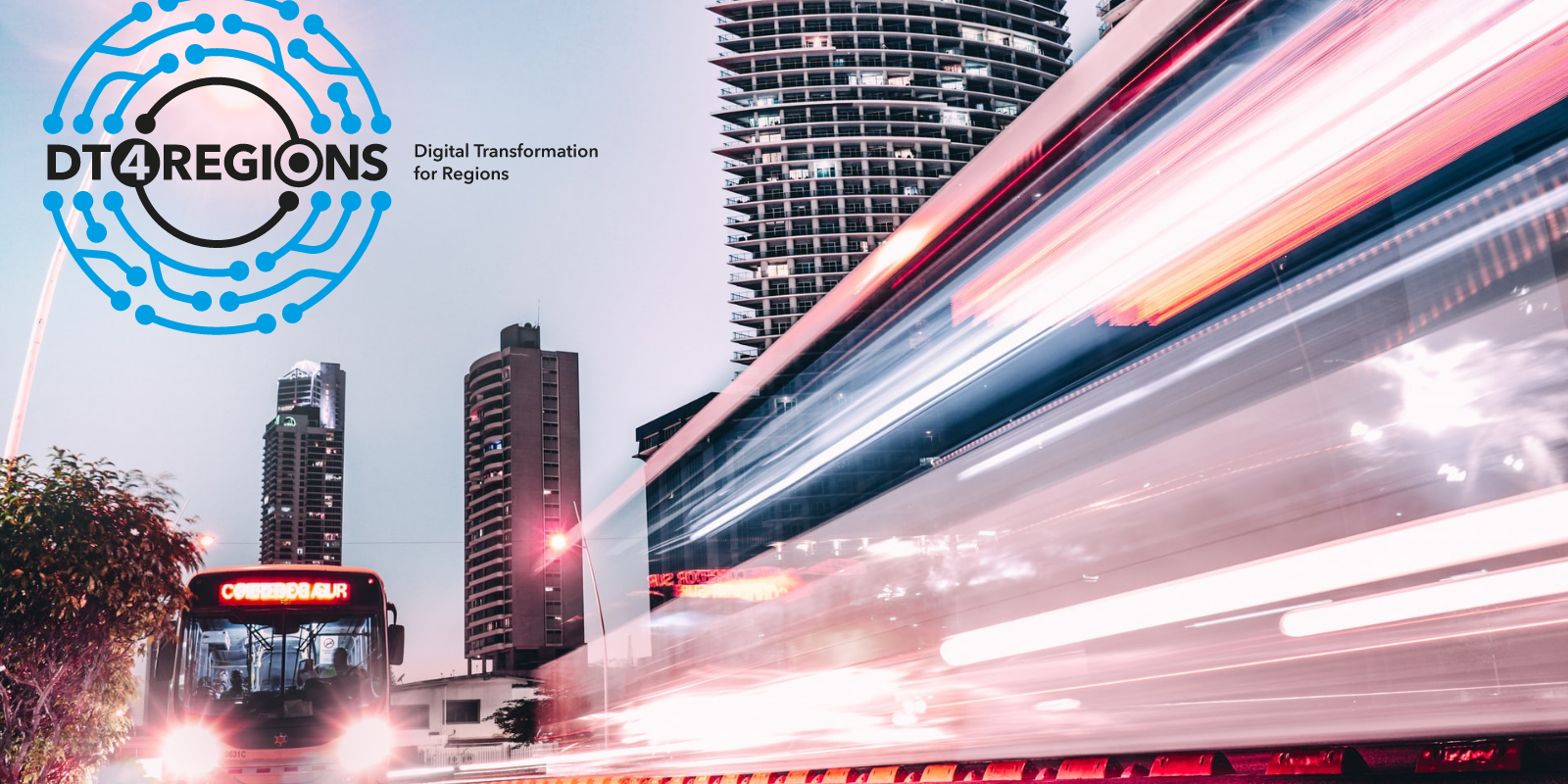
The proposed solution exploits the potential of the clustering techniques to help the Emilia-Romagna Region get insights into the mobility of the collaborators who commute to work and find homogenous areas of workers, in terms of their home locations and distance to their workplace.
Tactical Objectives
By identifying the location of workstations that yields the lowest travel times and costs, the solution proposed aims to
- reduce emissions produced by workers who commute to their assigned offices by private cars,
- enhance the work-life balance of the workers, especially those who live far from their current workplaces,
- generate a more efficient use of buildings and other working facilities to reduce energy and space consumption.
Methods
The proposed solution is a three-step process. First, we obtained the location (latitude, longitude) of the employees' homes and the workplace buildings. Secondly, we calculated the distance and driving time between the employee’s home and workplace. Based on our policy on smart working permits, we selected only employees more than 15 km away from the assigned workplace, and we performed a cluster analysis with the DBSCAN algorithm to create groups of employees based on their density within the regional territory. Finally, we also simulated a reassignment to the closest workplace building for grouped users and calculated the costs and emissions associated with each employee before and after re-assignment. What-if scenarios have been generated, and the effect of re-allocation in terms of time, costs, and emissions has been measured with a certain degree of approximation. In order to deliver results to managers and decision-makers, we propose an interactive dashboard to explore where the employees live and how many kilometers they have to travel to work, where they work, and where they potentially can work (smart) and measure the effect by simulation. The dashboard also offers a drill-through analysis to support the planning phase of searching for co-working places. The implementation details can be found in the repository linked in the resources (work in progress).
Functional requirements
Data scientists and geoprocessing experts are involved in carrying out the project in about 6 months.
Results and Impact assessment
Assuming that employees are allowed to work remotely for 49% of the workdays in a year, we estimate a 30% reduction in costs and emissions related to employees who travel to their assigned office. The individual average daily travel time would go from around 40 minutes to 12 minutes, saving about 70 hours per year. Based on internal studies on travel expenses (0.40 € / Km) and co2 emissions (138 g / Km) incurred by employees, the annual savings would be around € 1000 per capita, and the amount of CO2 saved would be about 2000 tons. In a further development, we aim to estimate the efficiency of buildings and make more analyses to optimize the usage of spaces.
Dependencies and constraints
The data on employees is pseudonymised to comply with the GDPR. The source code and all libraries used to create the solution are free-to-use and released with an open-source license, with the exception of the EgeCo georeferencing proprietary software (our internal geoprocessing database service). The critical aspect of the solution is the lack of information regarding the means of transport used by employees and the availability of workstations within the workplaces. Considering these limitations, costs and emissions are calculated supposing all employees go to work by private car and this is not a realistic picture and is overestimated. On the other hand, if the availability of workstations is known, it is possible to improve the simulation phase by assigning employees to new workstations by introducing constraints on the saturation of workplaces. Dashboard and data contain personally identifiable information of employees and are not available for public access.